It would definitely be interesting to explore the overlaps between the SSNA (which is made from qualitatively coded data) and the DeLab results here (where terms are based upon existing words in articles).
Question for @kristof_gyodi – how did you select the terms for analysis? (e.g “ensur_complian”, “effic”)? The list is not a cluster (there are no relationships between the terms in the list, necessarily), it is just the terms that co-occur with that top term most frequently, correct?
There are a lot of similar codes that we could compare – and see if the clusters look different (ours is a network of co-occurrences, so a bit different). But I’ll show you some of ours and see if you think the comparative would be of interest.
Let’s look at your term “covid-19_pandem”
here’s where our COVID-19 code is in the co-occurrence network:
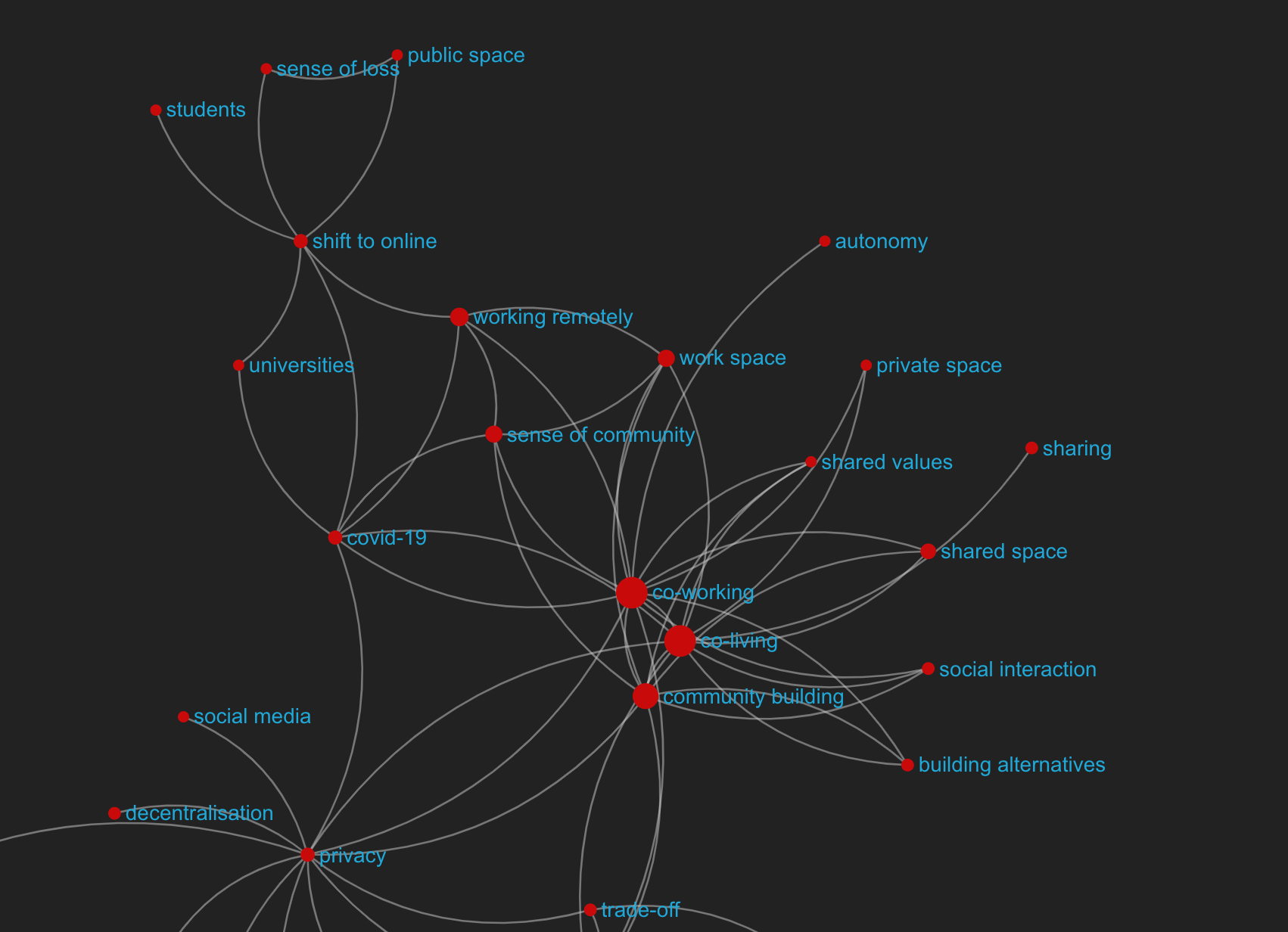
and here it is in its own ego network, with lower-level co-occurrences filtered out, leaving only the codes that most frequently co-occur with COVID-19
(so, perhaps a better comparison to your data):
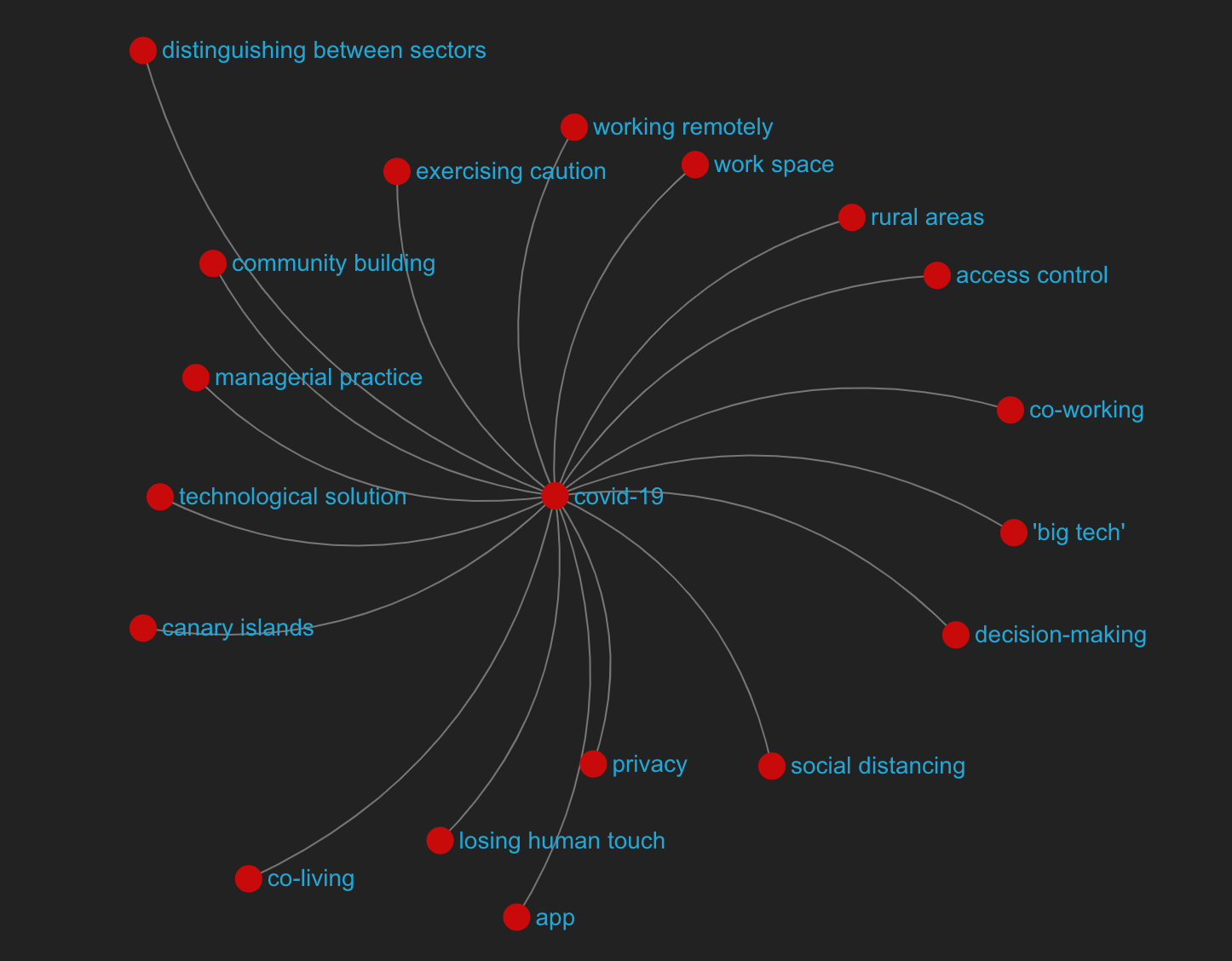
When we look at the ego network, we can see some overlaps with your data:
-
social distancing
, perhaps unsurprisingly. This concept is connected to social distance itself and its impacts – in the ego network, you can see codes like losing human touch
and working remotely
(and responses to the negative effects of those impacts, like co-working
, co-living
and community-building
). These could relate to your term “particip”, potentially. I also am guessing that your term “earlier this” might refer to people making comparisons to how things were before the pandemic (though hard to say), which relates to the concept of “rapid_chang”.
Let’s turn to that one, “rapid_chang”. We have the code adapting to new circumstances
, which is related. Here’s that ego network:
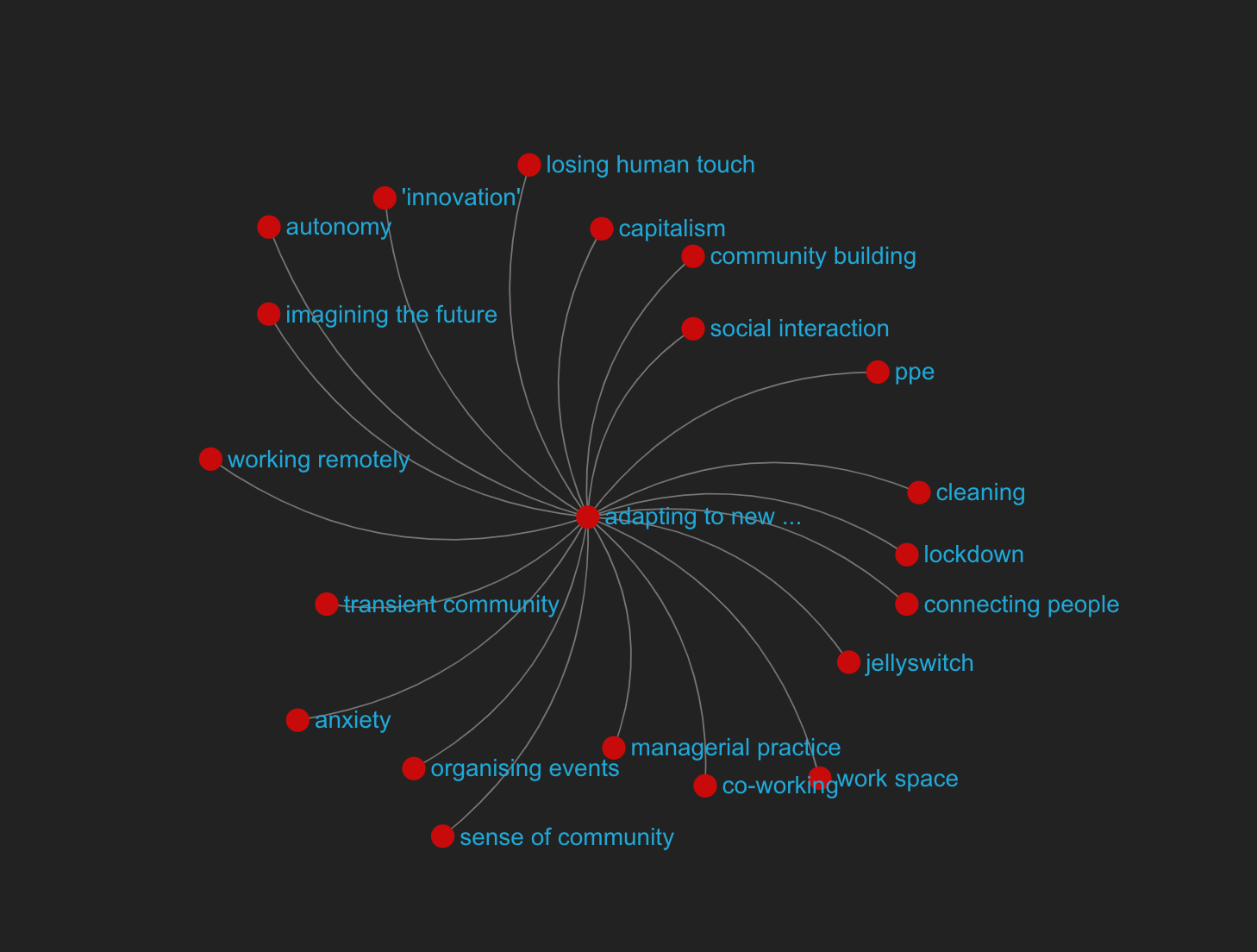
I see overlaps here, too. “collabor”, I assume is related to collaboration, a response to rapid change. We have codes like connecting people
, organising events
, and community building
(to create a sense of community
) as ways of adapting to new circumstances(related to your “new normal”). resilience
is a code we have as well, just outside of this co-occurrence level, that I know is increasing further as we are coding now (you can see anxiety
in ours, too, so there is a sense of having to keep it together for increasing lengths of time in the face of uncertainty
, another code).
We also have working remotely
, like your “remot_work.” I imagine “mandatori” has to do something with the required safety measures like lockdown
, ppe
, and cleaning
.
Your “rapid change” seems to refer to two things: the pace of technological change (digital transformation, app) and covid-19 related change. Heading back to the co-occurrence network, we can see these two kinds of change being discussed by our participants, too. imagining alternatives
is connected to tech adaptation
(not unlike your “digital transformation”) and app
.
Uploading: Screenshot 2020-10-20 at 12.07.35.png…
I’m also interested to see what the addition of the sentiment analysis does, and see if the theory we have about negative/positive meaning usually being clear contextually bears out in practice when we compare (/if adding any element of sentiment analysis way down the road makes any sense for us – we talked about this at one Masters of Networks with @melancon and one of the LaBri students). If I recall correctly, when we saw the sentiment analysis his student did applied to one of the OpenCare threads, it didn’t accurately capture the nuance – but that was a different method of course.
Interested to see how we could make further comparisons going forward! I’d love to understand how you theorise why the co-occurrences exist (how you explain why terms are connected, from the perspective of the people making the connections, and explanation of the social phenomenon giving rise to them). I’m always interested in how to move from the co-occurrences to explaining what gave rise connections themselves/what story they tell.